Subscribe to the podcast on your mobile device:
Apple Podcasts
Spotify
Stitcher
Google Podcasts
YouTube
iHeartRadio
Anyone who has any experience around precision agriculture is familiar with colorful maps that distinguish variability in a field. These maps may express vegetation greenness, crop canopy temperature, soil electrical conductivity (EC), or soil organic matter. But how are these maps produced? Where is the data measured? What sensors collect the data and how do they work? This episode of the FarmBits podcast features Dr. Trenton Franz, an Associate Professor and Associate Director of Research in the School of Natural Resources at the University of Nebraska - Lincoln. Trenton is trained as a hydrogeophysicist, and his research focuses on quantifying soil spatial variability for improving water management efficiency in agricultural production. In his research, Trenton uses a variety of non-contact sensors including neutron and gamma ray sesnors that measure soil properties related to nutrient and water movement. While these sensors may sound like science-fiction instruments, they have actually been used since the 1940s, although their usefulness in agricultural applications is only beginning to be understood. This is one of the challenges that Dr. Trenton Franz is addressing through his research - how can the measurements from these sensors be correlated to useful soil properties that can practically inform decision making for farmers. Trenton will discuss this challenge, the sensors that enable his research, the mathematics behind producing accurate soil property maps, and the properties that most impact crop productivity throughout this episode. Ultimately, this episode will connect the dots between soil property measurements, soil spatial variability maps, and management zones to demonstrate how we get from measurements to maps.
Opinions expressed on FarmBits are solely those of the guest(s) or host(s) and not the University of Nebraska-Lincoln.
On this episode
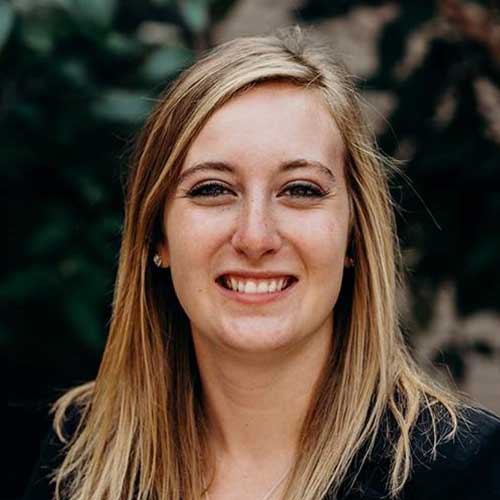
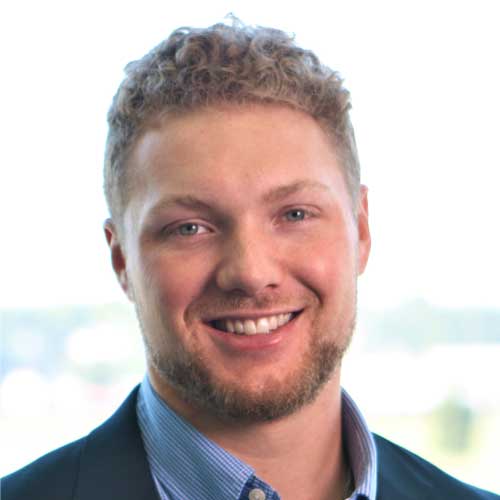
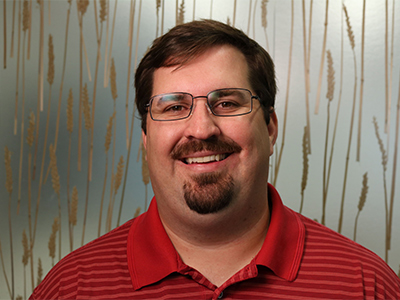
Show Notes
Trenton Franz Contact Information:
Twitter: https://twitter.com/tfranz7070
Website: https://snr.unl.edu/aboutus/who/people/faculty-member.aspx?pid=1906
Resources:
ESAP Model Sampling Software: https://www.ars.usda.gov/pacific-west-area/riverside-ca/agricultural-water-efficiency-and-salinity-research-unit/docs/model/esap-model/
FastTIMES - Engineering and Environmental Geophysics Society Applied Journal: https://www.eegs.org/fasttimes
FastTimes - Agricultural Geophysics Special Issue: https://app.box.com/s/ids60kr94z19esi48ultsg7gj3iamjtx
FarmBits Team Contact Info:
E-Mail: farmbits@unl.edu
Twitter: https://twitter.com/NEDigitalAg
Samantha's Twitter: https://twitter.com/SamanthaTeten
Jackson's Twitter: https://twitter.com/jstansell87
Read Transcript
Samantha: Welcome back to the FarmBits podcast for our third episode in the quantifying soil spatial variability series and our 10th episode overall. It is hard for us to believe that it is already the 10th episode.
Jackson: No kidding, in today's episode we're going to feature Dr. Trenton Franz, associate professor in the school of natural resources at the University of Nebraska-Lincoln. Trenton will tell you a little bit more about his background, specifically educationally, during the episode but his specialty is in hydrogeophysics.
Samantha: In practice, his research focuses on measuring spatial soil properties to better understand how water moves in agroecosystems and how we can use this knowledge to improve water management in agriculture.
Jackson: Through his research, he has extensive experience with using instruments to map soil properties such as soil moisture content, organic matter and other variables that matter to crop production. Samantha: In this episode, we are focusing on how measurements get turned into maps and what these maps can be used for. Jackson: Trenton will describe measurement technologies, how the data collected has turned into maps and what the value proposition of this information is for farmers.
Samantha: So, with that introduction let's jump into our discussion with Trenton. Thanks for joining us. Do you want to start off by telling us a little bit about your background and what got you started working in this field and in agriculture?
Trenton: Yep so I grew up in Colorado, kind of extended families in the water area a lot of water engineers in my family. Some real extended relatives in agriculture in Eastern Colorado. But nothing immediate in my family you know. After that I went on to University of Wyoming, where I played football and got degrees in civil engineering then did my PhD work after that at Princeton in civil and environmental engineering, and then went on to do a postdoc at University of Arizona really where I got into this soil moisture mapping technology that we'll talk about later on. And so really really my background's in the water area, and then when I took a job at UNL you know became pretty clear that you know water and agriculture are so you know tied together. You know we use about 90 of our water in this state for agriculture so it seemed like a natural fit to move into that space.
Jackson: Sure that's really cool and I think I remember you telling me at one point in time, I'm talking previously you did a little bit of work in Africa as well during your your PhD research there at Princeton.
Trenton: Yep so I did all of my field work in Kenya as part of a water and ecology project. So I lived in a tent out on the Savannah working with the Maasai for you know six to eight weeks at a time, so that was some interesting field work there. So I don't miss living in a tent.
Jackson: So, your current research right now, like you mentioned, is working with soil properties to quantify soil moisture kind of in real time and working at this agriculture and water interface, and it's my understanding that you use some high-tech soil property measuring devices to help us better inform water management decisions and agricultural production in general. So, can you describe a little bit more of your research in detail for us right now here at the University of Nebraska?
Trenton: Really into geophysical sensors and remote sensing now we can use some of these new cool tools and technology to look at, you know water efficiency. So, I work with a lot of different technologies from electromagnetic induction, to neutron detection to gamma-ray detection and these instruments are nice because they have- a lot of them are passive sensors and non-contact and so that way you can really scale them up and map entire fields instead of you know sticking a probe in the ground and moving around you know 160 acres. We can drive these on vehicles or tow them behind tractors and that sort of thing. So, it really allows us to get to the scale that we need for agriculture.
Samantha: That's awesome, we've seen these, some of your fancy sensors in the truck. So. I know it's awesome to see maybe, we can share some pictures but. So, what do you think is the most important or some of the most important soil properties to measure when quantifying spatial variability?
Trenton: So really we have the you know the challenge that we can measure you know these geophysical properties you know like electromagnetic properties or neutron properties and the challenges that convert those into useful things in agriculture. So you know, it's nice to have you know measurements of soil moisture. But really what we're at is you know the properties that control flow of water and nutrients through soil and so we have to, you know, connect these state variables like soil moisture with the fluxes of water and nutrients and that's really the biggest challenges is making that connection. So for you know, irrigation scheduling things like quantifying, you know soil field capacity and soil wilting point are really critical for defining those irrigation trigger points of that particular patch of soil. So for that that, would be important too for irrigation on the you know sort of nutrient management side. You know things like organic matter, organic carbon those sorts of things are probably of most interest.
Samantha: I was going to add on to that- is it difficult sometimes to find like a correlation to an exact physical property? So, for example if whether it's just high levels of clay or if it's compaction I mean do you have to do several different managements to maybe differentiate those things or you know some of those different measurements that you're doing?
Trenton: Yep so for you know the different instruments that are most suited to a particular property, so for electromagnetic properties those are very tightly correlated to clay percent for the neutrons. Those are nice, those are directly correlated to the mass of hydrogen or soil moisture and for the gamma ray detection. Those are more about the sort of underlying geology of the system and so the next trick is then converting that into something useful. So it's kind of a kind of a puzzle where you have some of the pieces and not all of them. You're kind of trying to stitch it together to come up with a you know a useful picture of what's happening at the site. So it's, I guess that's why it's kind of fun to do is it's you know it's a real big challenge. You don't really have fully complete information to make these sort of you know inferences about these things.
Jackson: So it sounds like for a grower out there who's looking at using some of these technologies and maybe adding these property measurements to their repertoire- they're kind of a complementary set of data in addition to traditional soil sampling for them right? Because in order to get a good measurement of the actual nitrogen for example or phosphorus or potassium you have in a field this is just good additional information to complement what they may get off of a traditional soil sample. Is that kind of correct?
Trenton: Yep so that's kind of how I
view it is we you know we always will still do soil sampling, just because that's our ground truth. So kind of how I view it is you know is making that correlation between the soil properties that we get from the laboratory and what we get with the imagery. And so you know we can combine multiple layers. Another big one is you know elevation is really important to take into account, or you know drainage area for particular slopes. Those are also important and so we want as many different layers of data as possible to make that correlation and that connection. And once we have multiple layers of information we can start to use some of these more advanced statistical techniques like machine learning and AI to help build that correlation structure and make better predictions of soil properties everywhere. So, kind of how I view it is let's trade some of that soil sampling, we don't have to take as many locations, we can put that budget or time and effort into soil mapping and still get better results and hopefully, you know the same type of cost for that type of data.
Samantha: That's awesome, you've already mentioned a few of the on-the-go soil mapping technologies that you have. But can you maybe tell us if there's any more that you haven't mentioned so far and which of these technologies are gaining the most traction.
Trenton: Yep so a lot of companies out there, so Veris has been used a long time using electrical reactivity so that's going to be very similar to electromagnetics. So another company out there is a Dual EM which is widely used by Crop Metrics, Crop X. Soil Optics another company out there that uses a gamma ray technology and then also Tremble is using several of these techniques and then Smart Farmer is integrating some of these sensors right with the you know the planting of the the actual seeds. So yeah, so it's kind of the wild west out there of all these different techniques and we're trying to figure out kind of which one you know is the most useful and most cost effective. You know if you get some of these actionable decisions.
Jackson: So you mentioned a couple other sensors that you particularly used in your research including cosmic ray detector and the gamma ray detector stuff like that. Are those just emergent technologies that we're still trying to figure out what place they might have in measuring properties for ag?
Trenton: Yeah so, honestly these technologies have been used since the late 1940s and 1950s and some of the you know increases and electronics and costs of the sensors have come down and kind of you know trickled through you know various scientific disciplines. I think they're you know just starting to you know make it more widespread into agriculture as we kind of you know some of the technology you know of how we apply water or you know fertilizer catches up to some of these instruments. So I think now that we you know have the ability to apply water and fertilizer in these spatial management zones we now you know like catch up with what are some of the techniques to get that underlying data that we need to optimize that process. Jackson: Yeah absolutely, so so when these technologies are measuring soil properties- what we're getting back on the other end is point data and that's basically measurements that are taken at certain locations within a field but maybe not be continuously measured throughout the field and so the distance between these points can vary laterally and longitudinally which kind of creates a different data resolution for each different data layer that we might get from these mapping technologies. And I think we see the same with soil sampling some people do zonal soil sampling, some people do grid soil sampling. So when you have point data like this how can you actually take this data and turn it into a continuous map that describes spatial variability across an entire field that folks can use practically in their operations? Trenton: Yep that's that's a you know a great practical as well as sort of you know theoretical question of how we do this. You know what I call upscaling of these soil properties- so you know one thing that you know I've dug a lot of holes in my life as part of the research one thing I found is that you know if you were say you know sample within a field you know 10 point data with inside let's say a 10 by 10 meter grid you would have about as much variability between those point samples and that 10 by 10 meter grid average is if you took 10 by 10 meter grids and then upscale that to your entire you know 65 hectares or 160 hectares. So there's just a ton of variability that exists at that point scale when you aggregate it up to sort of you know the you know management zone scale. So the nice thing about the geophysics is often these are the way the sensor works is it's integrating over some of those smaller distances for us so you know how far an EM wave propagates how far do you know neutrons or gamma rays mix is it's doing that averaging for us that's a small scale and so that's really, really what you know the advantage of these instruments is- we don't get that fine scale variability that you get from point samples and so that makes it a lead a little easier to you know upscale that process and fill in the parts of the map. The challenge is that when you compare a point sensor to sort of the you know this 10 meter scale you have a lot of you know variability still. So your correlation may only be 50 or 70 percent because it's just hard to match up those you know very fine scale what's sort of this intermediate scale. So, it's a mouthful there but I think it kind of makes sense when you start thinking thinking about these things you know practically and going out there and actually doing some sampling.
Samantha: So, building on this discussion on point data- can you potentially explain for our listeners what interpolation means and what are some potential issues associated with interpolation?
Trenton: Yep so this is basically you know how we take you know a series of points in an area and we how we fill in those missing pieces of data and so you know you want to build basically this the structure of you know how correlated your points are versus how far apart they are so that's called a variogram and so you can use that relationship between distance between your measurements and how quarter they are to do that filling in of that information and so the challenge of that is you need about you know 100 to 120 points in order for that relationship to work you know that's a lot of soil sampling in order to make that work. And so instead what we like to do is you know build that correlation between maybe 10 or 20 point samples between the geophysics and then use that geophysical map to help fill in that interpolation. So getting a little technical would probably call that co-creating or this in the term here basically you find that regression creating excuse me that's the term I'm looking for. So, you build that regression and then you add in that a relationship between how correlated points are as you increase that separation distance.
Jackson: Very cool yes, I know Sam and I we took a class on soil spatial variability last spring and did a little bit of kriging but certainly not to the extent that you had to go out and take 120 soil samples.
Samantha: No and then if you're doing it the two and a half acre grid which is pretty you know normal for people that's a large area of land or like I said a lot of samples.
Jackson: So how much does that sampling resolution- Sam just just kind of mentioned the two and a half acre grids, impact the outcome of creating an interpolation. So if you had a one acre grid sample versus a two and a half acre grid sample versus maybe a five eight sample how much does that effect the outcome?
Trenton: Yep, so I mean I think you know you'll probably get the same average value once you get to about you know 20 or 30 points inside of your area versus 60 you should get a very similar average value. It's really going to be sort of that you know standard deviation or that variability is what the part that you're going to miss you know, so it really depends on you know how are you managing your field are you doing it you know one uniform treatment of water and fertilizer then it's probably not going to matter that much. But if you're breaking your field into you know two degree sort of pie slices then yeah you're going to want to capture that variability. So it really you know is you know the sampling has to go with how you're going to actually implement that management and what you want to invest in and how do you get a return on that and that's really the big challenges is you know what is you know practical and pragmatic for management versus what is that underlying variability in your field and that's you know what we are trying to figure out, so.
Samantha: It's also dependent a lot on what you're trying to measure correct? So like organic matter may not vary as much as nitrate for instance.
Trenton: Yeah exactly so all these things you know they have a you know a natural distribution of you know how the you know the sediment was laid down, how it was weathered and then how you've managed it over the years and so you know that's a big challenge you kind of need some of that history in order to figure out what is that sort of pattern of variability and so that's kind of like we like the geophysics is that kind of you know a quick run of that. You can kind of tell what that underlying structure is and kind of what that you know basic picture looks like by running over with the geophysics.
Jackson: So, for somebody who's out there that's taking these soil samples or running the Dual EM, running the Veris- What are some practical steps that they can take to ensure the best possible quality data is going into that kriging and interpolation procedure for the best possible outcomes?
Trenton: Yep so in an ideal world, you would go out and you would you know do a geophysical map say with a Dual EM or Veris you kind of look at that as well as an elevation map and you could pick your sampling locations based off of sort of you know what those two maps look like. So, some USDA soil salinity lab has a nice piece of software things called ESAP where you can put in those layers and they'll actually pick smart sampling locations for you. Often though we don't have that luxury we're kind of doing that sort of you know we sample and then we do the geophysics or sort of a mixture of that and so that that's the challenge is you know kind of matching these things together. But you know I think we can get away with fewer soil samplings in the field if we do do smart sampling so that's kind of been my experience is you know we can probably reduce that by you know half or 75 percent by including some of these geophysical maps.
Samantha: So, as you mentioned I think a couple questions ago all this data only matters for how we're applying it or how we're using it, so what are these maps being useful for in terms of annual decision making or long-term decision making or what are you seeing a lot of people how they're applying it.
Trenton: Yep, so I think you know crop metrics is you know one of the leaders in this area initially if you know how do you take this imagery and turn it into you know irrigation scheduling as well as you know planting density you know if you want to vary your seed population. There's some algorithms they've developed for that in addition you know I think you know the gamma ray gives you a little bit different information to get more some of the soil chemical property information some more of the you know what Veris is getting you the organic matter and getting in more to some of the nitrogen management. So, I think you know it used for sort of all of the above and it's still kind of you know unknown what the optimal way to use this that data is. It kind of depends on the company and you know you know who's using you know this data set so there's no sort of you know right prescribed equation right now that we're using so.
Jackson: Sure, and so since this is kind of an emerging area of research- what have you seen to be the most effective soil property layers so far for characterizing spatial variability say as it relates to yield within a field?
Trenton: Yep so what I've found, we have a new publication on this is really that you know elevation is probably the primary controlling factor followed by some measure of soil variability, whether that be through you know Veris, Dual EM or soil moisture mapping. We kind of find that as a secondary factor. So, the nice thing about that is you know elevation data is pretty much widely available, freely available through Lidar or your you know your combine or your planner typically you can pull those layers off. And so that's a very you know low hanging fruit out there is to look at your elevation data also remote sensing. So if you uh you know have some of these tools you can get at you know things like landsat imagery and compute uh things like peak growing season greenness as we found is a as a pretty good metric. And so, it's kind of and again that's freely available data and then also your previous year's yield maps are going to be your best predictor of next year's yield so it's kind of a mixture of those different layers together so. Elevations great, previous year's yield and then if you want to get more to the remote sensing products some of the Landstat archive is pretty nice to get into that. I think that's awesome that you mentioned a bunch of like freely available resources or things that farmers already have at their disposal they don't have to go necessarily buy a really fancy sensor so that's awesome. Trenton: I fully utilize the free stuff and then after that see what you need you know.
Samantha: Yeah which one did you ask the spatial layers and then you want me to ask them. Jackson: I guess I was gonna kind of follow up Trenton, I was watching one of your presentations here recently I think it was from the Cosmos conference in Germany that she said when we were preparing for this this podcast and you mentioned the technique of basically synthesizing multiple different soil property layers into kind of a single explanatory variable I think that the acronym for that was EOF which you mentioned is similar to like a principal component analysis- could you explain a little bit about how that works and how that is able to combine multiple soil spatial variability layers into something that is explanatory of yield? Trenton: Yep so we'll get real nerdy here into some linear alpha unfortunately so hopefully we won't scare off too many of your listeners. But yeah, so this is a nice technique where you have the same layer that you collect multiple times over the year or many years and this technique basically allows you to split that spatial temporal data into spatial and temporal components separately and so it's a little different from principal component analysis which basically aligns it into your principal axes but it's not a space and time component necessarily. So, the upshot is that is basically is you can remove all the time varying effects from these data sets so Veris and Dual EM any of these things are gonna be affected by things like soil temperature. So, a moisture on the day that you collect that property and so this statistical techniques allows you to filter out those time varying components and just focus on what the spatial patterns are. So again, that's a mouthful but basically how we're using is we're trying to distill basically what is the underlying spatial properties from this sort of data that can vary from day to day and depending on you know what time of year that you collect it.
Jackson: I think that makes a lot of sense to us because you know we do a lot of work with imagery and imagery can change week to week that some of those underlying soil properties are going to be influencing how that crop performs in certain areas of the field and it sounds like that technique basically narrows it down to what are those consistent underlying properties that we're worried about.
Trenton: And same thing with historical yield maps, we've used this on you know if you have four or five yield maps of you know corn or soybean the same crop is you can really identify your underlying spatial variability of what your yield looks like. And so, it's a that's probably the easiest way to make management zones. My opinion is to take some of those historical yield maps and then run it through a technique like this to try and really find those you know like zones that sort of work as a single unit. So clustering is kind of another way to do that but I think this is - it sounds complicated but it's actually very straightforward when you run it through a statistical package.
Samantha: That's awesome and you led us right into what we want to discuss next. So we do talk about management zones a lot in our research just because it does apply to that specific management it can vary your nutrient management or your planting. So how does the information that you're collecting or how does this information help us to better select the variables we use to generate management zones? Which I think is kind of funny. If you want to elaborate on how the different variables are used to generate management zones.
Trenton: Yep, so again historical yield that seems like the no-brainer to use if you're you know looking at yield is that's obviously gonna be your best you know variable if you have that. Nice thing if you don't have historical yield for whatever reason is remote sensing can help fill in some of that so there's some nice uh this greenness index that I mentioned is a nice one for that's very correlated to a yield that they found through you know various studies. So, you can sort of recreate some of that historical yield if you don't actually have it. So, first thing you're going to kind of, how many pick how many management zones you want you know if you're comfortable with you know three five or seven is that can really help sort of define you know the algorithm and the constraints on that clustering algorithm. So that would be kind of the first thing is you know what can I feasibly you know manage for this field is it three zones is it five is a seven. And that will really help constrain you know that statistical algorithm. So, you know from you know talking to various farmers it seems like three to five zones is kind of the you know the sweet spot right now. Once you're getting more into that you get more into automation and just too time-consuming and it's pretty challenging to manage. So, yeah I think you can you know run some of these clustering techniques you know if you ahead of time pick you know three to five zones and that will really narrow down kind of what those management zones look like and whether or not they sort of look reasonable you know if you're trying to manage the same thing you know three different spots in your field is that's not very helpful so you kind of have to you know pick contiguous zones where you can actually feasibly manage that with your equipment. Samantha: So, this might be getting out of the scope a little bit of what we want to talk about but a previous graduate student in our lab did some research on how different fields have different variables that influence management zones more so like if a field is really hilly it elevation means a lot more than a field that's flat which seems very you know intuitive but do you have like some ways to test like you know which ones you want to use in your clustering? Trenton: Yep no that's a great question. So, I mean I think some of this comes to sort of you know basic field knowledge. You know that's coming from the producers is you know you know we only do so much with you know the statistics but it's really that deep knowledge that you know the producers have that you want to build into those management zones and have the you know the remote sensing and the geophysics helps supplement that is kind of what I would say. So, you know you know this field is you know rocky in certain spots you're going to have to build that information in because you know we're probably going to see it with the geophysics but you really want to you know sort of constrain you know how do you how do you make that algorithm work based off of your local knowledge, I think it's critical so.
Samantha: That's an awesome response we've been told that before we said, hand a farmer a map of their field and have them draw the management zones for you.
Jackson: I'm just sitting here thinking about that, it's super interesting because we want to approach a lot of these problems with the data with the math and try to make everything consistent and fit every single field but the reality is that there probably will always be a human component to what we're doing. And experience because we're I guess we're just not going to be able to collect enough data over enough years to get there. Yeah the real world's messy you know dirty, you know soil as you call it. Jackson: You can't call it dirt don't worry. Okay so what are some variables I know we've talked about a lot of variables so far but are there any variables out there that you believe are either undervalued, underutilized or that you're really looking forward to testing for yourself to see how they may impact crop performance or water use metrics.
Trenton: Yeah some variables in terms like what techniques we're using or like uh you know the organic matter that kind of variable I guess what do you what do you mean by that? Jackson: Sure so I guess.
Samantha: I think this one is on like the variables like organic matter and the next one can be on techniques.
Jackson: Okay yeah, I just don't know if there's one that is basically. I guess what I'm getting at is is there a variable that's particularly hard to measure or that we're just starting to be able to really measure for the first time that you're excited about that may have an influence on crops? Trenton: Yeah, no so my primary area is soil moisture so that's one that we've you know struggled with to get spatial information at sort of the right scale for agriculture. So you know we have great soil moisture products from remote sensing that are you know 10 kilometers so that's not very useful for most you know agricultural applications and we have point sensors that you know measure you know the size of a you know a basketball. So also pretty challenging so with the cosmic ray sensor we're able to get you know sort of 20 to 30 meter resolution data or soil moisture over the top foot or 30 centimeters and so we're excited about sort of that you know new scale that we're able to quantify. So, you know all of the sort of you know theory and modeling tells us that you know soil moisture and how it changes over time in space should be you know the best predictor of evapotranspiration. And then crop yield and so we're excited about you know making that connection between soil moisture and the history of soil moisture and what's going to happen with the yield. So it's still ongoing research and pretty challenging but I. think that's you know really uh you know what which really gets me excited is making that better connection between soil moisture and crop yield.
Samantha: What about nitrates because our research is in nitrogen so we're just really curious? Trenton: Right yep now unfortunately I didn't do well in environmental chemistry so yeah not the best person to ask that but kind of how I view it is if we get the water piece right in the soil then we have a chance of getting the nitrogen piece right in the soil because you really need that flow of water and how it's connected to the nitrogen cycle and carbon cycle really in order to pin that down. So, I'm definitely not you know an expert in that. I kind of stop with the the movement of water through the soils and then let you know folks like yourself figure out the nitrogen piece. So I think we can do a better job of mapping organic matter and organic carbon in the soil and I think that is going to be a you know a critical piece for helping resolve some of those some of those issues. But again that's that's not my primary area so.
Jackson: Yeah it's that's really interesting you know we've we deal with nitrate leaching a lot here in Nebraska and obviously nitrate is probably the chemical within the soil that moves the most similarly to how water moves in the soil profile. So, I definitely agree that your assessment of understanding water is the first step to understanding nitrogen for us. Trenton: I mean example like we map certain fields you know multiple times and we know where the wet parts of the fields are consistently wet over and over again you know it's probably going to be in a depression or some other zone like that or some type of soil property. So, we know that those are going to be the highest potential for nitrogen leaching is where you have those wetter spots of the field that are consistently wet over time. So that would be you know sort of where I would would start sampling is where you have these you know consistently wet spots in the field.
Samantha: Awesome so keep up the good work then the way our research gets better.
Jackson: And hopefully we can count on somebody else to figure out the
on the go nitrate mapping to make our lives a little bit more right.
Samantha: So what techniques and technologies do you believe are on the horizon to play a role in the future for shaping how we interpret soil property data and draw inferences from that data that was a little mouthful.
Trenton: Yep so.really what I'm excited about is you know what we call opportunistic sensing and so this is where we're gonna put some of these technologies embedded right on the farm equipment you know we're talking about landscapes maybe on you know a mail truck or some type maybe a you know a train moving through the landscape. So, we do this all the time and you know commercial aircraft we put sensors on those things and we use that data in our weather models that's why can we do the same thing you know in farming, or you know regional hydrology and so you know it's a big challenge you know to work with you know these you know private industry or or individuals. I think there's you know some opportunities through co-ops or other companies to get some of these you know sensors on you know communal sprayers or detasslers or something like that so we can collect these data sets. You know if we're collecting at scale and the cost comes down to something that's very reasonable as well these sensors are you know pretty expensive up front they last a long time
but you know that initial upfront cost is pretty challenging. So I think we have to do some sort of you know community sharing of those initial costs though.
Jackson: So, what does that system look like I guess one one question I have in my mind right now is: number one how often do these properties need to be mapped or is it just something that needs to be mapped once and then you're good to go? Because I think that would be one question I would have as far as that system you know do they need to be put on a planter every five years for example in order to to keep accurate data coming in.
Trenton: Yep no, great question what we found is you know for running this EOF technique here mentioned that we need about three or five maps at different water temperatures or soil temperatures and then that really distills down what the underlying you know spatial soil properties are. So, and those aren't going to change greatly over time you know on the order of you know probably decades depending on your management. So yeah so you know if you put on one sprayer maybe after two or three years you'd have enough data sets to run this analysis and you wouldn't have to to redo that or if you had more targeted excuse me approaches where you had you know you know so yeah a private company somebody with an ATV or a truck you could you know map the same sort of you know three by three mile region you know over the course of a season and get sort of that. You know, chunk
mapped out kind of kind of like how they do airborne lidar surveys in the state. They kind of do it in these tiles and I imagine something similar you could you know just start mapping out these tiles and eventually cover the entire state.
Samantha: Before we get to the last question I think we may have one more too based on that. But anyway, one thing that we found with like you mentioned earlier the elevation data from like different equipment right and like some combines and stuff and we look at that data and sometimes it really varies in quality are there other challenges with some of these sensors whether that's the RTK like GPS or other things that may be causing that quality to not be very good.
Trenton: Yeah you know so for you know GPS that's you know has to do with your ground stations and sort of your correction factors that you apply. You know honestly what we found is sort of the relative value you know is good enough so as long as the sensor is consistent with itself or the mapping period you just work with the relative elevation data not you know maybe the absolute value so. For elevation it's nice they have completed a a statewide lidar survey the USGS has that data so you know gotta be a little bit savvy to go out and clip out that data off the web but those data sets are out there through these different clearing houses. But that is a bit of a challenge to sometimes get access to that in your correct system.
Jackson: And just I guess going back one more question I just want to follow up on this piggybacking idea again. With piggybacking you mentioned it's good to get the maps at different soil temperature and moisture levels in order to kind of I guess span the range of soil conditions out there. Is it feasible you think within a typical agricultural year agricultural year to collect a data set that does span those different temperature and soil moisture regimes say with you know tillage, planting, spraying, harvesting and then maybe even a dry fertilizer application would you be able to capture all the different regimes that you would want?
Trenton: Yeah, I think so I mean we're probably gonna miss the really wet end obviously because you're not gonna be driving over those wet soils because you know you might get stuck out there. So, you'll kind of get the medium to sort of dry conditions which is probably pretty, good enough. You know most of the you know properties we care about are sort of in there in that you know medium sort of range at least for irrigation. Um obviously we want the wet pattern if we're talking about leaching and you know fluxes of water but you know it's just not really possible to collect that information you know with the vehicle so. But usually, you know if you collect you know five or six times we've found that you know we get three or four different very images from that just over the course of the year. So yeah, you can be strategic about when you sample out there but you know usually you know you'll probably find a different day if you go out you know three or four days later than we have except for maybe right now or a pretty serious drought but you know usually we have some sort of you know a different regime after you have a rainfall event. So yeah.
Jackson: So how close do these sensors need to be to the soil surface in order to actually make these measurements because I'm thinking there are a lot of people out there in Nebraska that have pivots that you know maybe this could be a sensor you could throw on a pivot and run around dry and maybe get a good measurement when that soil is one of those high moisture regimes. Trenton: Yep no that's a great idea you know I mean the cost is a little bit high for that but in terms of research, we've put a couple of comic ray sensors on pivots and done dry runs which is pretty cool to do you know a six-hour revolution to get that that measurement. So yeah, I know the patent recently came out where they're putting GPR on actual center pivots and so they're doing ground penetrating radar as the pivot rotates around. So, I think there's a lot you know opportunity of what kind of sensors that we can use that existing infrastructure on. So, I've seen GPR, IRT sensors mounted on pivots. So, I think it's kind of you know there's a lot of opportunities out there what kind of you know technologies we can put on these center pivots to use that as our you know basic infrastructure.
Samantha: Yeah, You just named a lot of acronyms is there like a great resource for people that can go to maybe see what different sensors are out there and what each of them measure?
Trenton: Yeah, I don't know if there's sort of a one definitive one that I can think of. You know it's kind of these companies that I mentioned kind of go into some more of the sort of techniques and how they're using it but yeah that's. A good one that might be a good resource to build I don't know if there's like a extension article on you know sort of geophysical techniques that are out there. So I know the uh the journal FastTimes. It's a society of- SAJEEP is the acronym but they're they're a more applied a journal for industry that goes into some of these techniques so that might be one location the FastTimes journal which has some more you know readable articles for a general audience compared to the journal articles. I can send you guys that link.
Samantha: So, we asked this at all our interviews- what is one piece of advice you have for our listeners out there who are working to understand variability in their fields or interpret soil property data to use for helping them improve their agricultural production?
Trenton: Yep so I think it's you know work smarter not harder you know you know we if you use your basic knowledge or some of these geophysical layers I think you can do you know smarter sampling to really reduce some of that workload. So, you know the you know two and a half acre grid sampling is kind of a you know a first start but I think you can you know really improve that by you know using some of these layers or your general knowledge to help sort of you know reduce some of that that labor that goes into that.
Samantha: Thank you so much to Dr. Trenton Franz for joining us today on the FarmBits podcast.
Jackson: His knowledge on soil sensing technology, mapping techniques and soil moisture data collection really demonstrated the importance of measuring and understanding spatial soil data. Samantha: I thought Trenton did an excellent job of explaining how the data processing methods work and how they can be applied to get practical benefit from soil spatial variability measurements.
Jackson: Yeah, absolutely I also thought his explanations were really clear and they really made complex topics easier to understand. My favorite aspect of this episode was Trenton's discussion of why spatial variability has to be separated from time variability if we really want to understand the underlying variability within a field oh and I also really enjoyed his discussion of how these sensors can be piggybacked onto other implements and machinery during typical farm operations to make even better use of that equipment for spatial soil sensing.
Samantha: Absolutely it was very interesting, so next week we will talk with Cory Willness of SWAT maps about how they use spatial data to characterize management zones.
Transcripts are generated using a combination of speech recognition software and human transcribers, and may contain errors. Please check the audio before quoting in print and write farmbits@unl.edu to report any errors.